In WS 2023/24, the seminar will not be held.
Machine Learning and Uncertainty Quantification for Physics-Based Models WS 2022/2023
Quick Info
Time and Place
Offered in Winter Semester
Time: Thursdays 1400-1600
Place: 5502.EG.234
Regular presence is required
Program
MSc.
Language: English
3 ECTS, 2 SE
Links
Module Handbook
Registration
Course Calendar
Moodle
Literature
List of seminar papers will be given at the Kick-off meeting on 20.10.2022.
Exam
Type: presentation
Time and Place: during regular class times
Grade Breakdown
Grading is pass/fail. Requirements to pass: active participation, paper presentation, handout/slides submission
What's this course about?
Machine learning and uncertainty quantification (UQ) are ubiquitous in modern science and engineering applications. In the last two decades, UQ for complex physical processes has been developed rapidly with a focus on grid-based process models such as finite element models which are well established in engineering applications. On the other hand, machine learning techniques have not traditionally been applied to physics-based models. The recent surge in data-driven models based on machine learning techniques such as deep learning is changing the computational science and engineering landscape. Novel hybrid models based on neural networks are emerging and are already enhancing traditional methods. In this seminar, we discuss theoretical and computational aspects that arise from combining PDE-based models and neural networks, in particular, physics-informed neural networks (PINNs), neural networks for PDE approximation, and applications in UQ and turbulence models.
How does it work?

SELECT A PAPER
Given a list of papers select the one most interesting to you or propose your own paper (relevant to the Seminar's topic). All course material (papers, schedule etc.) will be uploaded to Moodle.

Questions
For any questions the lecturers and TAs are available to you during office hours. Prior email appointment is required.

PAPER PRESENTATION
Present the paper to your fellow students. Engage in a joint discussion after presentations.
Meet your teachers
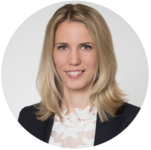
Lecturer
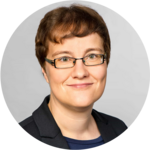
Lecturer
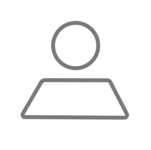
TA
Dr. Leila Taghizadeh